Try asking someone working in the data field ‘What’s the difference between a Machine Learning Engineer, a Data Scientist, a Data Engineer and a Data Analyst?’
Compare the answers and I’m sure you’ll notice the following:
- There is no clear answer
- All roles overlap one another
- Some responsibilities can be handled by them all
And most importantly you did not understand (and that’s ok! 😄) So let’s make sure you get an answer by the end of this blog.
The blog is composed of five sections:
- Section 1 focuses on the role of a Data Scientist
- Section 2 focuses on the role of a Machine Learning Engineer
- Section 3 focuses on the role of a Data Engineer
- Section 4 focuses on the role of a Data Analyst
- Section 5 explains the collaboration between the four roles
Let’s dive into each section.
1. Who’s a Data Scientist?
A Data Scientist’s primary job is the extraction of the data, while also drawing meaningful insights from it. This role focuses on the data related tasks: extracting, cleaning, transforming, and visualizing data to derive valuable information from it. They can also use this data to build predictive models for testing purposes.
Another minor, but important responsibility of a Data Scientist is data storytelling where they communicate insights through interactive dashboards in the context of a story using business intelligence tools.
2. Who’s a Machine Learning Engineer?
A Machine Learning Engineer’s primary role is to recognize hidden patterns to teach machines how to perform on unseen data. They take the models prepared by the data scientists and deploy them to production.
This role focuses on the modeling related tasks: training, testing, comparing and fine-tuning models to deploy them into production at scale.
In addition, Machine Learning Engineers oversee the models performance meticulously, ensuring that these models adapt and evolve with the dynamic data landscape. When deviations in performance are noted, or when new data patterns emerge, these engineers are responsible for retraining models, ensuring they continue to deliver precise and reliable outputs.
3. Who’s a Data Engineer?
A Data Engineer’s primary job is to prepare and structure data for analytical or operational uses. They are typically responsible for building data pipelines to bring together information from different source systems.
Data engineers often work as part of an analytics team alongside data scientists. The engineers provide data in usable formats to the data scientists and Machine Learning Engineers who run queries and algorithms against the information for predictive analytics, machine learning, and data mining applications.
They also deliver aggregated data to business executives, analysts, and other end users so they can analyze it and apply the results to improving business operations.
4. Who’s a Data Analyst?
A data analyst is primarily involved in collecting, processing, and performing statistical analyses of data. Their main role is to help companies make informed business decisions by providing data-backed insights, all while coming up with insights from the data.
Data Analysts are usually the ones responsible for reporting data-related findings to others in their team, be it technical or non-technical members. By utilizing storytelling techniques and clearly visualizing data through graphs and dashboards, they come up with creative and simple ways to explain their findings.
They also introduce data-driven solutions to specific business problems with the results from their analysis, improving business processes and performance.
5. Where does the confusion come from?
Although each role has its own title and responsibilities, it’s better to see the big picture where Data Scientists, Machine Learning Engineers, Data Engineers and Data Analysts work hand-in-hand on a project.
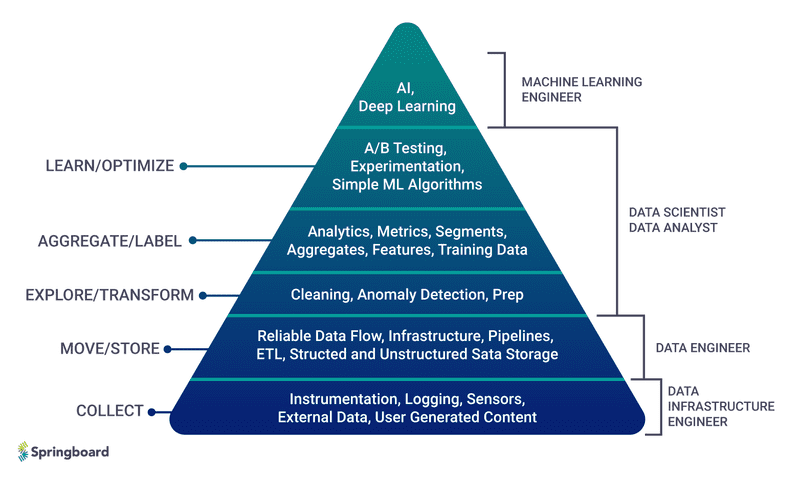
Data Scientists and Data Engineers often collaborate to achieve complex tasks with seamless efficiency. For instance, Data Engineers are tasked with establishing a robust pipeline for data collection, integrating databases, and APIs to ensure the smooth inflow of data. In parallel, Data Scientists focus on setting data requirements, ensuring data quality, and preprocessing the data provided by the Data Engineer.
Furthermore, Data Analysts are instrumental in the process of hypothesis testing. Collaborating with Data Scientists, they design experiments, analyze key metrics, and interpret results, providing insights into customer behaviors and preferences that are shaped by changes in the products or services.
In scenarios where a Data Scientist develops a machine learning model for experimental purposes using the data procured by the Data Engineer, the model is then escalated to a Machine Learning Engineer. Machine Learning Engineers play a critical role in ensuring that the models are not only optimized but also integrated seamlessly into applications, enhancing their reliability and scalability. They focus on perfecting the models to ensure they are efficient and reliable in a live environment.
Each role, though distinct, collaboratively contributes to the creation of a comprehensive solution that underscores the team’s collective efficiency and innovation. Their united efforts are geared towards the realization of a solution that encapsulates the team’s shared goals and objectives, and that’s what matters.
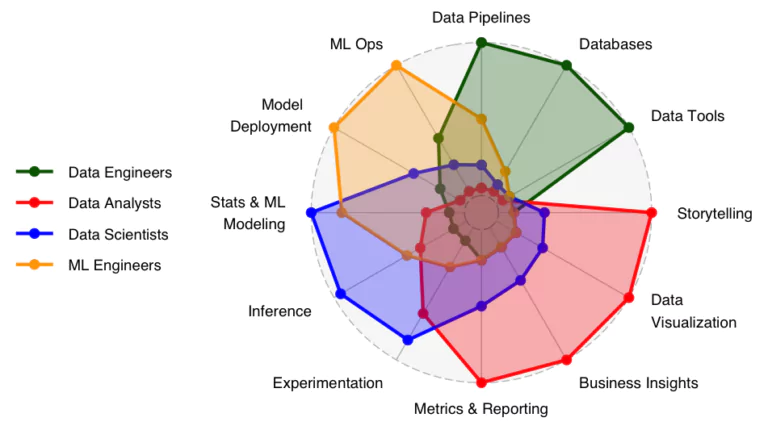
At the end of the day, you’ll choose one role and be in direct contact with the others. Along the way you’ll be exchanging knowledge, contributing in one phase and learning in the other.